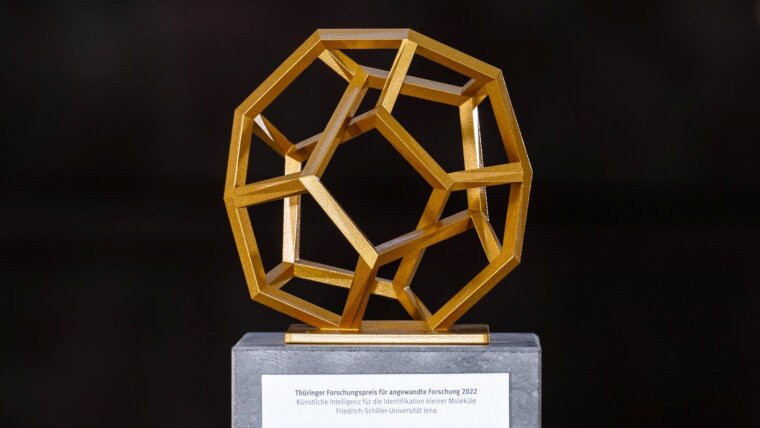
- Life
Published: | By: Ute Schönfelder
Source article
A bioinformatics research team from Friedrich Schiller University Jena has won the 2022 Thuringian Research Prize for applied research, Thuringia’s Science Minister Wolfgang Tiefensee announced today (06 April) in a video presentation. The prize of 12,500 euros, awarded for the development of machine learning methods for identifying small molecules, went to the team comprising Prof. Sebastian Böcker, Dr Kai Dührkop, Dr Markus Fleischauer, Dr Marcus Ludwig and Martin Hoffmann.
Prof. Dr Sebastian Böcker, Chair of Bioinformatics, 3rd from left, holds the Thuringian Research Award 2022 in his hands. Dr Markus Fleischauer, left, Dr Kai Dührkop, 2nd from left, Dr Marcus Ludwig, 4th from left, and doctoral student Martin Hoffmann, right, were also honoured.
Image: Jens Meyer (University of Jena)Small molecules – called metabolites – are ubiquitous. Every living thing produces metabolites. They are synthesised and converted in the metabolism of every living cell. They serve as building blocks for cellular components, as energy stores or signalling substances. “For humans, they play a huge role as active substances,” Prof. Böcker explains. “New drugs are often variations of metabolites that occur in nature: moulds, for example, have shown us how penicillin can kill bacteria. This has saved the lives of many millions of people.”
However, identifying new active substances from nature and making them usable is time-consuming, costly and labour-intensive. “In addition, we often don’t even know which unknown molecular structures we’re actually looking for,” says Böcker. To detect small molecules in cell and tissue samples, for example from medicinal plants, we record a mass spectrum. In this process, the molecules are broken down into fragments and the mass of those fragments is determined. These results can then be compared with data from reference measurements to identify existing molecules. However, it’s clear that this method can only find molecules of which the structure is already known and recorded in a relevant database.
Machine learning assists in finding the matching structure
And this is precisely where the work of the Jena bioinformaticians comes in, and for which they have now received an award. They are developing methods that enable researchers to use mass spectrometry data to identify molecular structures for which no pure substances are available. These structures have also never been identified in nature. The researchers use machine learning methods for this purpose. With their search engine “CSI:FingerID”, they have developed a tool with which mass spectrometry data can be “translated” into information about the chemical structure – a molecular fingerprint – making it possible to determine those structures that best match the mass spectrometry data. As in a Google search, the result is a more or less extensive list of possible hits. The COSMIC method, based on this search engine and also developed by the bioinformaticians, additionally determines a score that evaluates the quality of the suggested top hit and deduces from that score whether it is correct or incorrect. This allows us to automatically select the most promising hits when screening thousands or even millions of candidates.
But what if the molecular fingerprint of the unknown substance can be determined by mass spectrometry but cannot be assigned to any previously known molecular structure? This also happens quite often. “There are more chemically feasible molecular structures than there are atoms in the universe,” says Böcker. But the bioinformatics researchers have also developed a solution for the numerous molecules whose structure cannot yet be found in any database in the world – the CANOPUS method. This method, also based on machine learning, assigns metabolites to a specific substance class without having to identify them exactly. “We can therefore determine whether it’s a sugar molecule, an amino acid, an alcohol or a bile acid, for example,” says Böcker. CANOPUS answers this question for more than 2,500 compound classes. In many cases, this information is sufficient to answer important biological or medical questions.
Molecular identification process accelerated
What all methods have in common is that they perform the analysis of measurement data, which would take a human being many weeks to years, in just a few hours. This not only speeds up the process of identifying previously unknown small molecules. It also allows much more data to be analysed, so that, for example, candidates for new drugs can be detected more quickly and efficiently. Researchers from all over the world use the methods developed by the Jena team many thousands of times a day – more than 200 million times in total so far. And the methods are not only used in academic research. The five prize-winning scientists founded a company several years ago that also transfers the methods to the business sector, thus making the research and development achievements of the University of Jena visible at national and international level.